
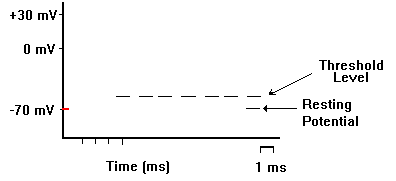
Simulate static synapses and study how excitation and inhibition affect the patterns in the neurons’ spiking outputĭefine mean- or fluctuation-driven regimes This feature of synapses in the brain is called Short-Term Plasticity and causes synapses to undergo Facilitation or Depression. Next, we will extend the model and model dynamic synapses – whose synaptic strength is dependent on the recent spike history: synapses can either progressively increase or decrease the size of their effects on the post-synaptic neuron, based on the recent firing rate of its presynaptic partners.
#ALL OR NONE PRINCIPLE OF NEURAL TRANSMISSION CODE#
In this tutorial, we will model chemical synaptic transmission and study some interesting effects produced by static synapses and dynamic synapses.įirst, we will start by writing code to simulate static synapses – whose weight is always fixed. Once the chemical diffuses across that space, it changes the permeability of the postsynaptic membrane, which may result in a positive or negative change in the membrane voltage. Instead, a spike in the presynaptic cell causes a chemical, or neurotransmitter, to be released into a small space between the neurons called the synaptic cleft. These synapses do not physically join neurons. In this tutorial, however, we will focus on chemical synapses, which are more common in the brain. Specialized electrical synapses make direct, physical connections between neurons. Synapses connect neurons into neural networks or circuits. Tutorial 3: Simultaneous fitting/regressionĮxample Model Project: the Train IllusionĮstimated timing of tutorial: 1 hour, 10 min Tutorial 4: Model-Based Reinforcement Learning Tutorial 2: Learning to Act: Multi-Armed Bandits Tutorial 2: Optimal Control for Continuous State Tutorial 1: Optimal Control for Discrete States Tutorial 1: Sequential Probability Ratio Testīonus Tutorial 4: The Kalman Filter, part 2īonus Tutorial 5: Expectation Maximization for spiking neurons Tutorial 2: Bayesian inference and decisions with continuous hidden state Tutorial 1: Bayes with a binary hidden state Tutorial 3: Synaptic transmission - Models of static and dynamic synapsesīonus Tutorial: Spike-timing dependent plasticity (STDP)īonus Tutorial: Extending the Wilson-Cowan Model Tutorial 1: The Leaky Integrate-and-Fire (LIF) Neuron Model Tutorial 3: Combining determinism and stochasticity Tutorial 3: Building and Evaluating Normative Encoding Modelsīonus Tutorial: Diving Deeper into Decoding & Encoding Tutorial 2: Convolutional Neural Networks Tutorial 4: Nonlinear Dimensionality Reduction Tutorial 3: Dimensionality Reduction & Reconstruction Tutorial 6: Model Selection: Cross-validation Tutorial 5: Model Selection: Bias-variance trade-off Tutorial 4: Multiple linear regression and polynomial regression Tutorial 3: Confidence intervals and bootstrapping Tutorial 1: Differentiation and Integration Prerequisites and preparatory materials for NMA Computational Neuroscienceīonus Tutorial: Discrete Dynamical Systems
